- 0 replies
- 85 views
- Add Reply
- 0 replies
- 519 views
- Add Reply
- 0 replies
- 1,058 views
- Add Reply
- 0 replies
- 1,273 views
- Add Reply
- 0 replies
- 2,342 views
- Add Reply
DEM2STREAM ArcGIS Desktop tool
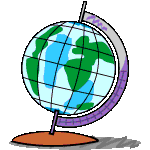
By yousef2233,
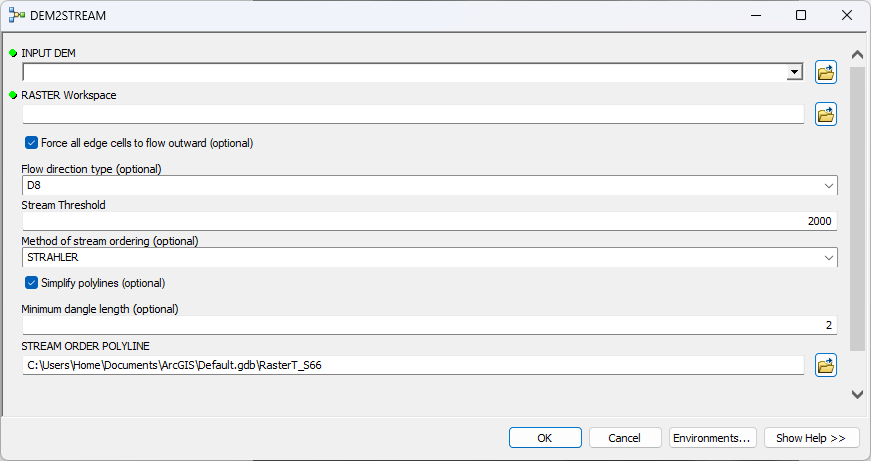
Hi everyone
This straightforward tool generates a stream order network from elevation data (DEM). The only input required is a DEM. As an open-source tool, it is accessible and easy to use.
If you encounter any issues, feel free to contact me at [email protected].
Tested with ArcGIS Desktop 10.8.1😊
Let me know if you'd like further refinements
Download Link
To create a stream network and determine its order in ArcGIS starting with a Digit
NASA Successfully Acquires GPS Signals on Moon
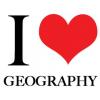
By Lurker,

NASA and the Italian Space Agency made history on March 3 when the Lunar GNSS Receiver Experiment (LuGRE) became the first technology demonstration to acquire and track Earth-based navigation signals on the Moon’s surface.
The LuGRE payload’s success in lunar orbit and on the surface indicates that signals from the GNSS (Global Navigation Satellite System) can be received and tracked at the Moon. These results mean NASA’s Artemis missions, or other exploration missions, could benefit from
GNSS spoofing in conflict zones disrupts wildlife tracking and hampers research and conservation efforts
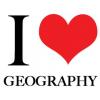
By Lurker,

In war and conflict zones, the jamming of Global Navigation Satellite System (GNNS) signals by military forces disrupts the tracking of tagged animals, and has increased in frequency following the recent escalation of conflicts in Eastern Europe and the Middle East. Such disruption to data collection strongly hampers research into the protection and conservation of endangered animals.
For decades, scientists have been uncovering the secrets of animal movements using various technological so
Before GPS There Was LORAN
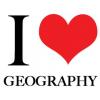
By Lurker,
LORAN — an acronym for Long Range Navigation — was a US byproduct of World War II and was similar in many ways to Britain’s Gee system. However, LORAN operated at lower frequencies to improve its range. It was instrumental in helping convoys cross the Atlantic and also found use in the Pacific theater.
How it Worked
The video shows a Loran-A receiver, which, in its day, would have been known as LORAN. The A was added after versions B and C appeared. Back in the 1940s, som
Hyperspectral imaging lidar system achieves remote plastic identification
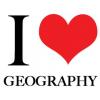
By Lurker,
Researchers have developed a new hyperspectral Raman imaging lidar system that can remotely detect and identify various types of plastics. This technology could help address the critical issue of plastic pollution in the ocean by providing better tools for monitoring and analysis.
"Plastic pollution poses a serious threat to marine ecosystems and human livelihoods, affecting industries like fisheries, tourism and shipping," said research team leader Toshihiro Somekawa from the Institute for
-
Forum Statistics
8.7k
Total Topics43.5k
Total Posts